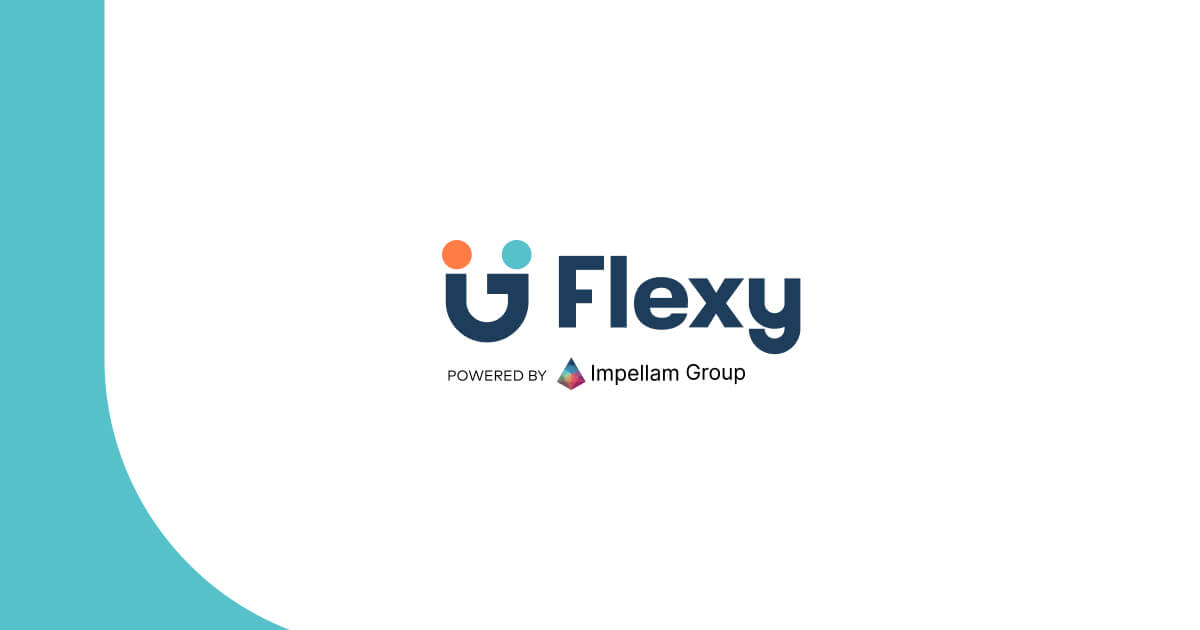
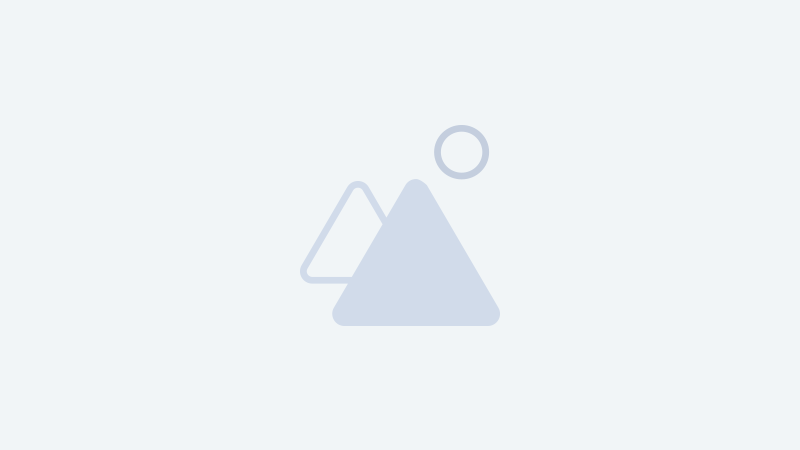
Author
2021-08-18
5 mins minutes
Providing transparency when using Artificial Intelligence to make decisions in recruitment
Providing transparency when using Artificial Intelligence to make decisions in recruitment
Given the daily barrage of articles that highlight the emergence of the age of Artificial Intelligence (AI), the announcement that Amazon has ditched AI recruitment tool is quite intriguing.
AI driven automation has been key to Amazon’s e-commerce dominance, be it inside warehouses or driving pricing decisions. It’s no wonder then that Amazon opted to apply AI in another mission-critical task, that of recruiting top talent. Apart from driving efficiencies through automation, AI is supposed to deliver data-driven objectivity in recruitment decision making process freed from human bias and prejudice. As put by a member of Amazon project team, “we literally wanted it to be an engine where one is going to give it 100 CVs, and it will spit out the top five, and we’ll hire those.”
The company’s experimental hiring tool used Artificial Intelligence to give candidates scores ranging from one to five stars – much as shoppers rate products on Amazon. Amazon’s computer models were trained to vet applicants by observing patterns in resumes submitted to the company over a 10-year period. Most came from men, a reflection of male dominance across the tech industry. In effect, Amazon’s system taught itself that male candidates were preferable.
Decades of research confirm the idea that human beings reason in two different ways. The work of Daniel Kahneman who, alongside collaborator Amos Tversky, pioneered the field that has come to be called behavioural economics, showed that we all have two modes of thinking, which he labelled System 1 and System 2. System 1 is fast, automatic, evolutionary ancient, and requires little effort; it’s closely associated with what we call intuition. System 2 is the opposite: slow, conscious, evolutionary recent, and a lot of work. System 1 is amazing, in short, but it’s also really buggy. It often takes shortcuts instead of reasoning something through thoroughly.
Going from Amazon’s endeavours, it’s obvious that AI-driven decision making processes are not a panacea for ending System 1 type prejudice. Although AI decision-making is often regarded as inherently objective, the data and processes that inform it can invisibly bake inequality into systems that are intended to be equitable. The idea that AI could function unaffected by bias reflects a misunderstanding of how the technology works. All machine intelligence is built upon training data that was, at some point, created by people. We see the data that goes in, the maths that goes in, and the results that come out. But how the decision was formulated is a black box.
At Flexy, we embrace machine learning in our decision making processes from predicting optimal hourly rate for jobs, to identifying the most suitable workers for a specific assignment. We do so, though, in full recognition of its capabilities and limitations.
In our approach, efficiencies are driven by enhancing user’s productivity and not from striving to replace human operator. Flexy assists employers to sift through hundreds of candidates by extrapolating key aspects of candidate’s profile against job requirements and scoring them accordingly. An ordered by suitability score list of candidates is presented to employer to choose from. By tapping on candidate’s profile, Flexy produces a visual representation of the output.
An unsupervised method that applies association rule mining on co-occurrence frequency data obtained from a corpus (list of job descriptions per job category offered by Flexy) allows for detecting key terms from job description as shown below (highlighted in Italics).
Office Receptionist/Administrator
If you are passionate about hospitality, have some relevant admin experience and are looking for an exciting and new opportunity, then we want to hear from you.
We are looking for candidates with previous reception/admin experience. Only applicants eligible to work in the UK or have a valid UK work permit/visa will be considered for the above reception position.
Manage the meeting room booking system, checking catering arrangements for the following day. Booking in external visitors. Booking all couriers, taxis and other logistics services as requested by the business. Successful candidate needs to be well versed in word, outlook and good at touch typing.
These terms (and their synonyms derived from word2vec), job type, job location and employer id are the input variables into our trained regression model and the output is the suitability score per eligible candidate for job (ranging in [0,1] where 1 is 100% suitability for job type).
Dataset used for training regression models (logistic regression in our case) consists of bias-free data points that carry information about candidate’s experience, personality traits, reliability as expressed by attendance in previous shifts, availability, distance from job and average ratings from employers for previous shifts.
Algorithm has learnt to assign different weights for data points per job type. For example, for a low skilled job where turning up on time and having the right attitude is important, the algorithm puts more emphasis to personality and attendance related data points.
Insights to drive workforce performance
Workforce insights in your inbox
Sign up for our newsletter with the latest workforce management news, insights, analysis and more.