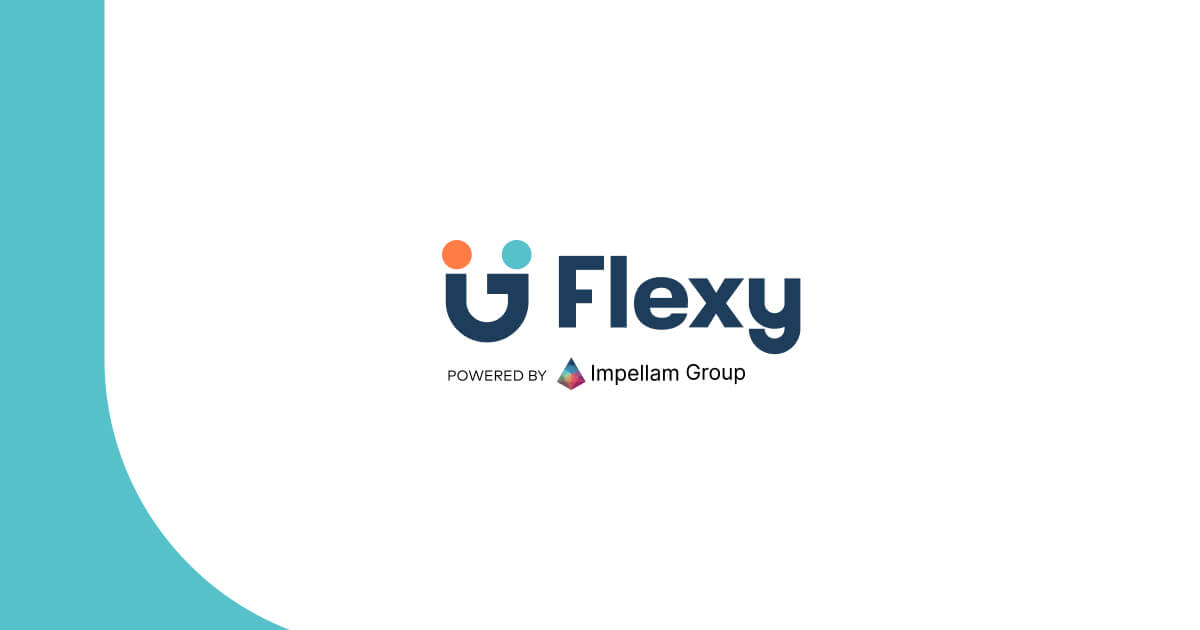
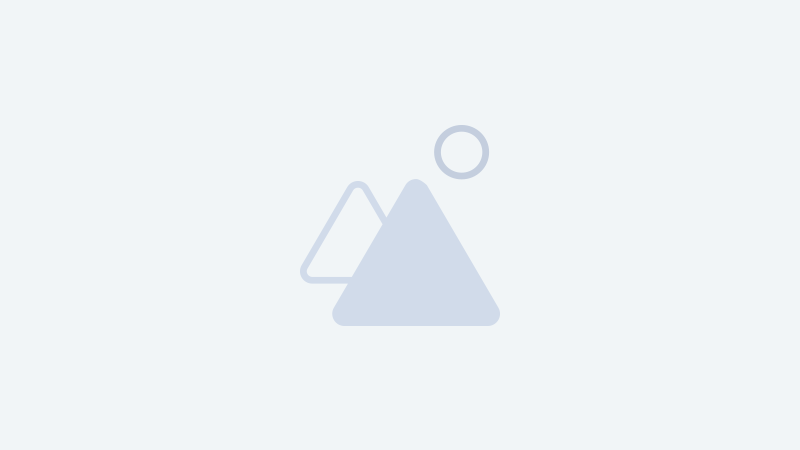
Author
2021-08-31
5 mins minutes
Solving business challenges with machine learning
Solving business challenges with machine learning
This is a guest post, by Digitalist Group. You can read the original article here – http://digitalistgroup.com/work/solving-business-challenges-with-machine-learning
In an era where technology evolves and changes rapidly, the temporary staffing industry has been rather slow to innovate. Relying on traditional employment agency methods often results in employers becoming frustrated with the lack of reliability, and poor-quality of temporary workers, and leaves temporary workers disillusioned by low pay and a mismatch of requirements.
Flexy is an inexpensive but efficient solution. By utilizing psychometric and forensic data analysis, Flexy matches the best candidates to the most suited jobs, maximising job satisfaction for workers, and in turn maximising productivity for the employer.
Employers utilise an online dashboard where they can post jobs, schedule shifts, review applications, and invite workers who have profiles that match their requirements. These jobs are then pushed onto an app on the worker’s phone, where workers can search and apply for jobs, and once accepted, submit timesheets for shifts they have worked.
The most compelling advantage of Flexy is efficiency, which is achieved through use of machine learning algorithms. Traditionally, employment decisions are fuelled by subjective information about the candidate; simply put, it comes down to how well candidates are perceived by a recruiter. A human recruiter collects information from the candidate regarding experience (via a CV), or personality (via an interview), or past performance (if available), then makes a decision based on that information.
Gaining better insight with machine learning
However, humans are not very efficient at processing information, especially when large amounts of highly dimensional and disparate data are involved (large number of candidates with several data points to consider).
Machine learning applied here can deliver unrivalled insights into selected candidates, employers’ preferences, or even industry idiosyncrasies.
While there are key data points that influence an employment decision, the importance of this information varies from job to job, and there is no one-size-fits-all formula. This is where machine learning truly excels, as it is able to adjust the importance of various data points for any particular job category, and over time, potentially even individual hirers.
For example, Flexy takes into account a number of attributes to determine candidate suitability for specific job including personality traits, experience, reliability and performance related metrics. These attributes contribute to candidate’s overall suitability score for the job. Nevertheless, employers may want to prioritise differently which attributes are more important for their workforce and job type. Instead of hardcoding the weighting on candidates’ attributes, Flexy’s search engine is capable of continuously learning weights on attributes from data employers generate in the system.
Utilising machine learning at Flexy
Digitalist Group is helping Flexy to customise matching algorithms per employer. On top of that we also train, deploy and evaluate a number of machine learning models (e.g. recommender systems, clustering and regression models) aimed at solving key business challenges including initiatives for reducing “No Shows” (individuals not turning up for their shifts) and real-time hourly rate suggestions based on job category, time and location. To do so, our Digitalist technical team designed a solution architecture capable of efficiently collecting and mining data on real-time basis, equipped with built-in mechanisms for evaluating new versions of machine learning algorithms with real customers by utilising A/B testing techniques.
UI concepts and visual language for customer touch points
Digitalist design team was tasked with delivering the UI concepts and visual language for all key customer touch points of Flexy service. The design concept was to have two distinct UI design approaches to delineate between the two target user groups; the companies that advertise for jobs and the workers who are seeking short-term work.
We introduced the idea of having a ‘warm’ UI for the desktop admin UI, where jobs are posted and a ‘fresh’ UI for the mobile app so that short-term work seekers can easily find and assess the suitability and desirability of a job posting on the go. What is important for this group is to be able to scan the jobs quickly. We identified that the worker demographic or job seekers are likely to be young, college leavers, university students or graduates therefore the UI reflects a fresh, modern, inviting look and feel.
The admin UI is where employers can furnish the posting with important data such as descriptions, the location, remuneration and contact information. It also gives the ability to view candidate profiles to assess their personality type, experience and skills as well as a number of other very useful functions such as shortlisting potential candidates. The sophisticated search engine is responsible for facilitating the matching of candidates with jobs and generating an algorithmically curated team.
Overall this clean design approach has embraced modern UI patterns to make the tasks for both audiences as efficient as possible. Last but not least, it was utterly important to deliver a design language capable of reassuring end users about machine-learnt decisions made at every step of the way and highlighting the great efficiencies offered by such approach.
Insights to drive workforce performance
Workforce insights in your inbox
Sign up for our newsletter with the latest workforce management news, insights, analysis and more.